How to Spot a Fake Online Review
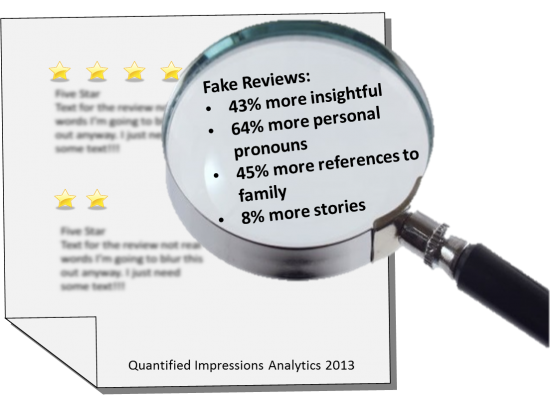
In last week’s blog post, we revealed whether positive or negative user reviews matter more to potential customers. This week, we turn our attention to deceptive reviews.
User reviews are incredibly important for businesses – a study from Cornell University found a movement of one point on Travelocity’s five-point scale changed a hotel room’s rate by an average of 11%. Given the swaying power of user reviews, the growing concern regarding how trustworthy reviews are is no surprise. Gartner, an IT research and advisory company, estimates that by 2014 between 10% and 15% of all online reviews will be fraudulent.
We decided to leverage our quantified communications platform to shed light on the differences between real and fake reviews. We started by analyzing a corpus of hotel reviews, put together by researchers at Cornell University, separated into solicited fake reviews and genuine reviews. We then used our natural language processing technology to analyze the linguistic differences between genuine and fake reviews in order to see if we could uncover the language signals that identify fake reviews.
After analyzing the data, we found that the language in fake reviews has the following differing characteristics:
- 43% more insight
- 64% more personal pronouns
- 45% more references to family
- 8% more stories
- 9% longer
What do these results indicate? A high percentage of references to family, high use of stories, and longer length indicated that the reviewer does not have experience with the product or service they are reviewing, so they compensate by talking about unrelated topics. Although personal pronouns are normally an indicator of honesty, in this context it may be the case that deceptive reviewers are focusing more on their personal opinions, while honest reviewers are focused more on the features of the product they are reviewing.
After running a meta-analysis, we found these results to be consistent with academic and scientific analysis. Researchers from Cornell University, using the same corpus of hotel reviews, discovered a positive relationship between deceptive reviews and imaginative writing. A study from Northwestern University, Deceptive Reviews: The Influential Tail, analyzed the language used in deceptive reviews and found that potential indicators of a fake review include a higher word count and increased reference to external factors, such as family.
In the largely unregulated realm of anonymous user reviews, quantified communications technology can help shed light on what’s genuine and what’s fake. This made us wonder: how do user review websites, like Yelp and Amazon.com, currently screen for fake reviews and are their processes effective? The answer may surprise you, and we will reveal it in next week’s post.