Can We Use Computational Social Science to Detect Fake News?
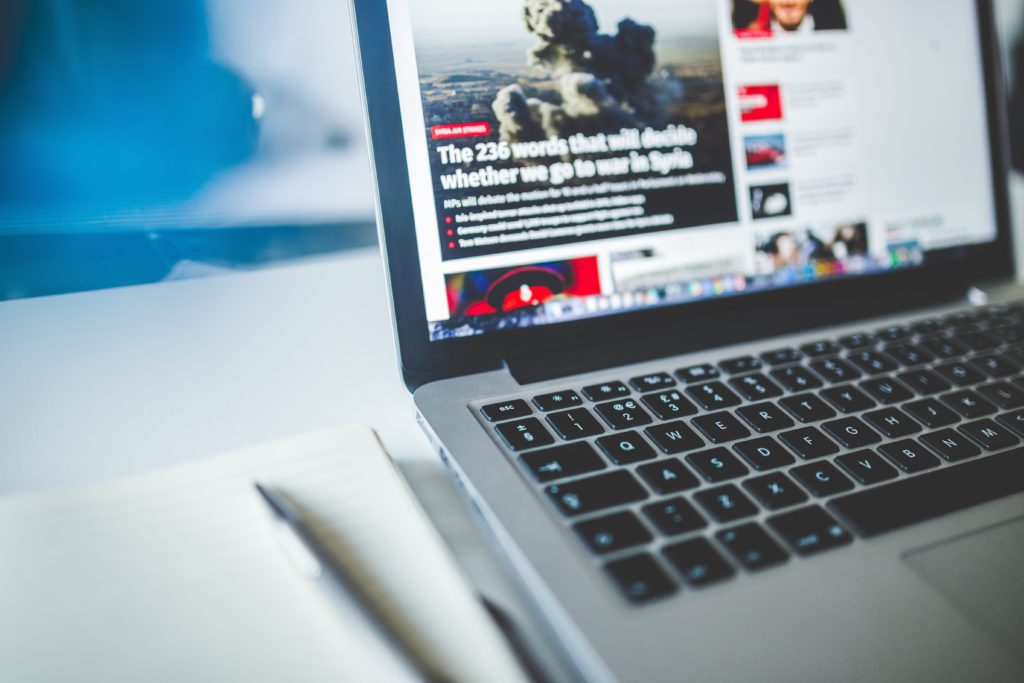
In case you hadn’t heard, 2020 is an election year. And that means we’ll be inundated with political news from all sides (as if we hadn’t been already). But in this “post-truth” world of fake news and alternative facts, how can we ensure that what we’re reading, believing, and sharing leading up to the election is real news?
Lately, we haven’t done a very good job. A recent New York Times analysis showed that, from January through October of last year, US Facebook users shared the top 100 fake political stories more than 2.3 million times, propelling those false stories to 158.9 million views and 8.9 million interactions. And the engagement with fake stories, according to the analysis, has increased along with the campaign fervor. And yet, campaign leaders on both sides of the aisle are failing to take a stand, claiming there’s only so much they can do to combat social networks’ minimal content restrictions.
So where does that leave the onus to detect, report, and shut down fake news? On individual citizens? On organizations like The Alethea Group, which views disinformation as a national security threat and works to protect campaigns from misinformation? On the social networks, themselves?
It’s a hotbed issue, for sure, but there’s one thing that’s certain: each of these organizations has the ability to do more than they know to stop the proliferation of fake news.
The Key Solution: Computational Social Science
A recent study out of University of Michigan, “Automatic Detection of Fake News,” leveraged two broad data sets of both fake and legitimate news to build fake news detection models that identified fake news with 75 percent accuracy.
In analyzing these data sets, the researchers found several significant indicators of fake versus legitimate news:
“[…] the language used to report legitimate content in the FakeNewsAMT dataset often includes words associated with cognitive processes such as insight and differentiation. In addition, legitimate content includes more function words (e.g., pronouns such as he, she), negations, and expressions of relativity. On the other hand, language used when reporting fake content uses more social and positive words, expresses more certainty and focuses on present and future actions. Moreover, the authors of fake news use more adverbs, verbs, and punctuation characters than the authors of legitimate news.
“Likewise, the results […] show noticeable differences among legitimate and fake content on the celebrity domain. Specifically, legitimate news in tabloid and entertainment magazines seem to use more first person pronouns, talk about time, and use positive emotion word, which interestingly were also found as markers of truth-tellers in previous work on deception detection. On the other hand, fake content in this domain has a predominant use of second person pronouns, negative emotion words and focus on the present.”
Here at Quantified, we’ve done a lot of work on the linguistic differences between truth and lies, and you can see the highlights in my TED-Ed lesson, but what’s more important here is that the researchers proved that we can use computational science to quickly and accurately suss out the alternative facts from the actual news and have encouraged researchers and practitioners to build upon their research to combat the proliferation of fake news.
“First, computational linguistics can aide in the process of identifying fake news in an automated manner well above the chance level. […] while linguistics features seem promising, we argue that future efforts on misinformation detection should not be limited to these and should also include meta features (e.g., number of links to and from an article, comments on the article), features from different modalities (e.g., the visual makeup of a website using computer vision approaches), and embrace the increasing potential of computational approaches to fact verification (Thorne et al., 2018). Thus, future work might want to explore how hybrid decision models consisting of both fact verification and data-driven machine learning judgments can be integrated. […] Finally, with the current investigation and dataset, we encourage the research community and practitioners to take on the challenge of tackling misinformation. The datasets introduced in this paper are publicly available at http://lit.eecs.umich.edu/downloads.html.”
No More Excuses
For too long, Internet giants, campaign leaders, and individual consumers have taken a passive approach to disinformation, and that’s enabled false information to spread at alarming rates, influencing voting behavior and other important decision making in the United States and abroad. But the truth is, our hands aren’t tied. With the power of computational social science, we can now take outcomes like fake news and actually measure their behavior patterns to discover the root inputs. This power is unlocking all sorts of new opportunities, from lie detection to fake news identification to influence analytics, and it means that we can no longer claim “there’s nothing we can do” about fake news. These innovations represent a fundamental shift in our ability to understand and evolve human behavior, and to take action against the misinformation we’ve been all too happy to spread online in recent years.